Xinran Li
Ph.D. candidate @ HKUST
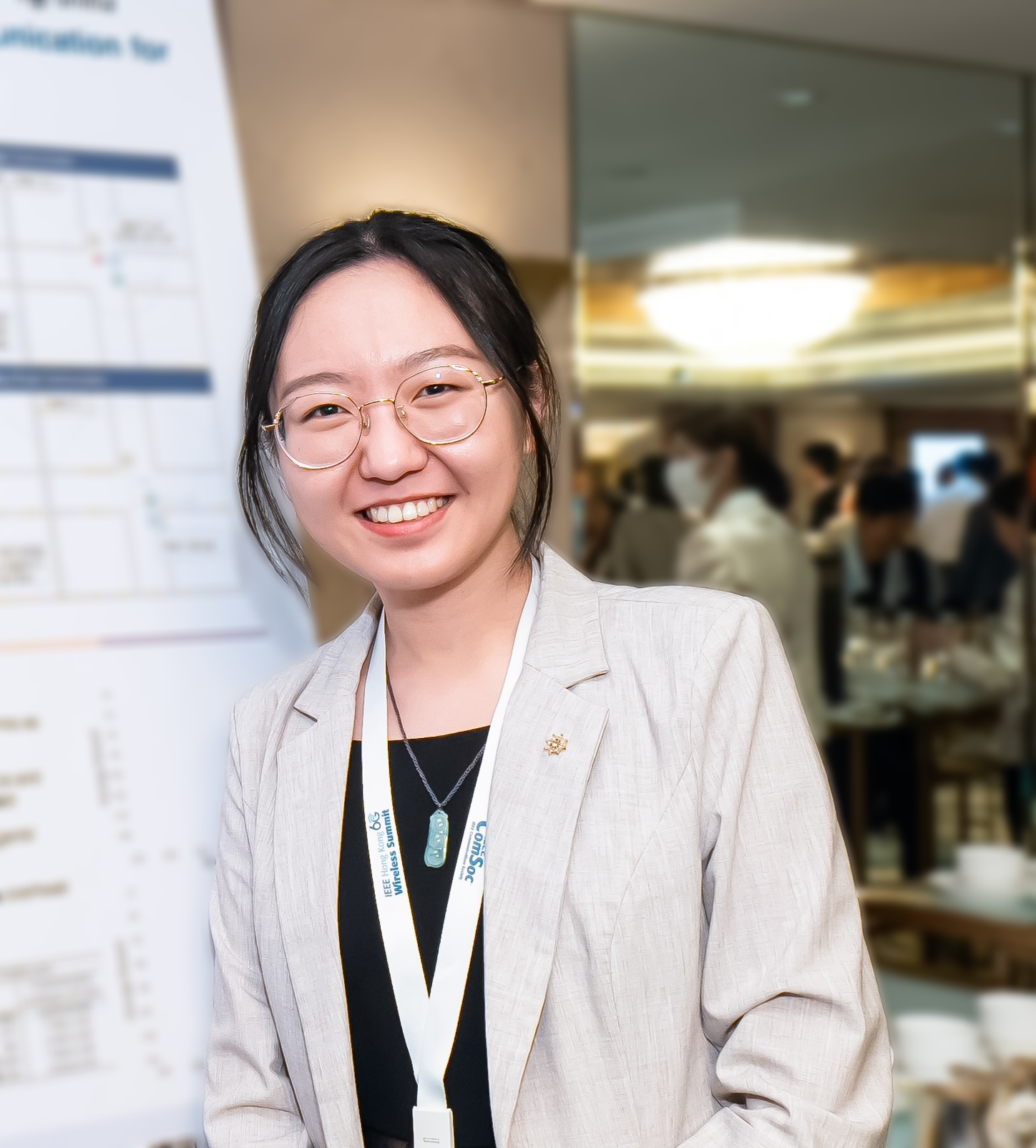
Hi there! I am a final-year Ph.D. student at Hong Kong University of Science and Technology (HKUST), working under the guidance of Prof. Jun Zhang.
Throughout my PhD study, I’ve had the privilege of collaborating closely with Prof. Ling Pan. I also conducted research internships with the embodied AI team at TeleAI, led by Dr. Chenjia Bai, and with AI Businesss, Alibaba, led by Mr. Qingguo Chen.
Prior to that, I received my Bachelor’s degree in Electronic and Information Engineering from Beijing Institute of Technology (BIT) in 2020. As part of my undergraduate experience, I spent time at the Australian National University (ANU) working on my honor thesis under the supervision of Prof. Salman Durrani in 2020. In 2021, I interned at the Department of Open Source Algorithm System at SenseTime, mentored by Dr. Wenwei Zhang and led by Dr. Kai Chen.
My research centers on decision-making problems, with a particular focus on multi-agent systems. I primarily leverage reinforcement learning and large language models as core methodologies to tackle these challenges.
You can find my CV here (Last updated: June 2025).
I expect to graduate in Spring 2026 and am actively seeking research positions in industry. I’m always open to discussions and potential collaborations! Feel free to reach out to me via email or wechat (lxr-rrr) if you have a potential role that might be a good fit, are interested in collaboration, or simply want to chat about research.
News
Jun 02, 2025 | Our paper, Learn as Individuals, Evolve as a Team: Multi-agent LLMs Adaptation in Embodied Environments, is posted on Arxiv. |
---|---|
Jun 01, 2025 | Our paper, Revisiting Multi-Agent World Modeling from a Diffusion-Inspired Perspective, is posted on Arxiv. |
May 01, 2025 | Our paper, C2IQL: Constraint-Conditioned Implicit Q-learning for Safe Offline Reinforcement Learning, has been accepted to ICML 2025! Congrats to Zifan! |
Apr 01, 2025 | I have joined AI Businesss, Alibaba as a research intern led by Mr. Qingguo Chen. |
Jan 24, 2025 | Our paper, Exponential Topology-enabled Scalable Communication in Multi-agent Reinforcement Learning, has been accepted to ICLR 2024! Big thanks to Prof. Xiaolu Wang, Dr. Chenjia Bai and Prof. Jun Zhang! |
Jan 22, 2025 | Our paper, Reinforcement Learning with Intrinsically Motivated Feedback Graph for Lost-sales Inventory Control, has been accepted to AISTATS 2025! Congrats to Zifan! |
Dec 12, 2024 | Our paper, Learn How to Query from Unlabeled Data Streams in Federated Learning, has been accepted to AAAI 2025! Congrats to Dr. Yuchang Sun! |
Sep 26, 2024 | Our paper, Kaleidoscope: Learnable Masks for Heterogeneous Multi-agent Reinforcement Learning, has been accepted to NeurIPS 2024! Big thanks to Prof. Ling Pan and Prof. Jun Zhang! |
Aug 05, 2024 | I have joined the embodied AI team led by Dr. Chenjia Bai as a research intern at TeleAI. |